Algorithmic accountability refers to the responsibility of developers, organizations, and policymakers to ensure that algorithms, especially those used in decision-making, are transparent, fair, and justifiable. As algorithms become increasingly prevalent in healthcare, finance, law enforcement, and beyond, algorithmic accountability has become crucial to maintaining trust, ensuring fairness, and protecting individuals from harm.
In this article, we will explore the concept of algorithmic accountability, why it matters, the key principles that govern it, the challenges in achieving it, and the role of governments, companies, and developers in promoting it.
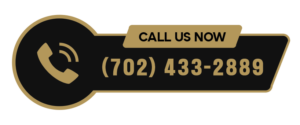
What is Algorithmic Accountability?
Definition and Key Concepts
Algorithmic accountability refers to the idea that the creators and users of algorithms must be held responsible for the outcomes of those algorithms. In this context, an algorithm is any set of rules or procedures followed by a computer system to perform a task, make predictions, or aid in decision-making.
Accountability ensures that algorithms operate in an understandable, ethical, and justifiable way. It is about answering the question: Who is responsible when algorithms cause harm, error, or unintended consequences?
Differentiating Between Algorithmic Accountability and Transparency
While both transparency and accountability are essential components of ethical algorithm use, they are distinct concepts:
- Transparency refers to the ability of external parties (e.g., consumers and regulators) to understand how an algorithm works, what data it uses, and the reasoning behind its decisions.
- Accountability, on the other hand, goes beyond transparency. It emphasizes responsibility for outcomes, even if those outcomes are not entirely predictable or transparent. This includes taking corrective actions when algorithms cause harm or fail to operate as expected.
In summary, while transparency allows stakeholders to understand algorithms, accountability ensures those algorithms remain responsible for their decisions.
The Role of Accountability in Algorithmic Decision-Making
Accountability is critical in preventing or mitigating harmful outcomes from algorithmic systems. Whether an algorithm is used to decide who gets a loan, predict recidivism in the criminal justice system, or determine medical treatment options, accountability ensures that the algorithm’s creators and the organizations that use them are answerable for the impact these decisions have on individuals.
Without accountability, algorithms may operate in opaque, biased, or discriminatory ways, potentially harming vulnerable populations.
The Rise of Algorithms in Decision-Making
How Algorithms Are Integrated into Various Sectors
In recent years, algorithms have become central to decision-making in various industries and sectors. Some key areas where algorithms play a significant role include:
- Healthcare: Algorithms are used in diagnostics, personalized medicine, and patient care predictions. For example, AI-based systems can help doctors identify diseases like cancer more accurately and quickly than human physicians.
- Finance: Credit scoring, loan approvals, and fraud detection systems rely on algorithms to make financial decisions. These algorithms evaluate a range of data to determine the likelihood of repayment or identify suspicious activity.
- Law Enforcement: Predictive policing tools use algorithms to forecast crime hotspots and allocate police resources. These systems can also be used in criminal sentencing, parole decisions, and risk assessments.
- Human Resources: Many companies use algorithmic systems for recruitment, resume screening, and performance evaluation. These systems are designed to identify the best candidates but can also perpetuate biases if not adequately monitored.
Examples of Algorithmic Applications and Their Impact
- Amazon’s Hiring Algorithms: A few years ago, Amazon developed an AI tool to help with recruitment, but the algorithm was biased against female candidates. It preferred male candidates for specific technical roles, highlighting the need for proper oversight in hiring algorithms.
- COMPAS in the Criminal Justice System: The Correctional Offender Management Profiling for Alternative Sanctions (COMPAS) system is used to assess the risk of reoffending. However, studies have shown that it may disproportionately rate Black defendants as higher risk, raising concerns about racial bias in algorithmic decisions.
- AI in Healthcare: Machine learning models have been used to predict patient outcomes, but if not correctly calibrated, they can perpetuate existing healthcare disparities. For example, an algorithm used in hospitals may underpredict the health risks for Black patients due to underrepresentation in the training data.
The Benefits and Risks Associated with Widespread Algorithm Use
The use of algorithms brings both significant benefits and risks. Some of the advantages include:
- Efficiency: Algorithms can process large volumes of data much faster than humans, leading to quicker decisions.
- Objectivity: When properly designed, algorithms can remove human biases from decision-making, leading to more consistent results.
- Cost-Effectiveness: Automation through algorithms can reduce the need for human labor, leading to cost savings for organizations.
However, the risks cannot be ignored:
- Bias: If an algorithm is trained on biased data, it will reproduce and amplify those biases.
- Lack of Accountability: Determining who is responsible when an algorithm causes harm can be challenging.
- Opacity: Many algorithms, especially those based on machine learning, are considered “black boxes,” meaning their decision-making processes are not easily understandable.
In the next section, we will explore why algorithmic accountability is essential for addressing these risks and ensuring the ethical use of algorithms.
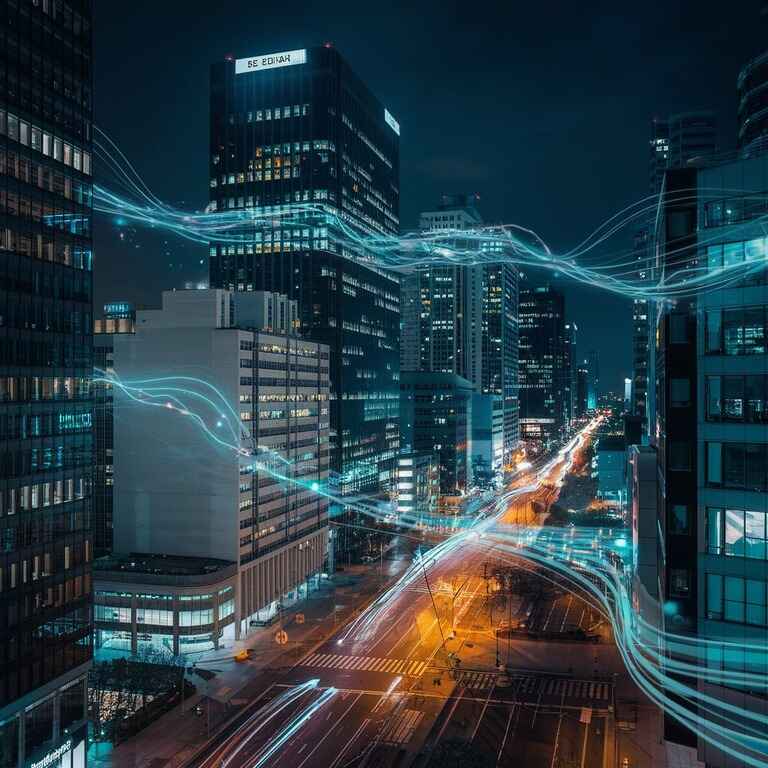
Why Algorithmic Accountability Matters
Ethical Implications of Algorithmic Decisions
The widespread use of algorithms in decision-making raises significant ethical concerns. Unlike human decision-makers, algorithms operate based on data and mathematical models, which can lead to consequences that may not be immediately apparent or ethically sound. Without accountability, the following ethical issues may arise:
- Discrimination: If not correctly designed or tested, algorithms may unintentionally perpetuate existing biases. For example, a hiring algorithm may favor candidates of a particular gender or race due to biased training data.
- Privacy Violations: Algorithms that process sensitive data—such as health or financial information—can lead to privacy breaches if not adequately secured or monitored.
- Autonomy and Control: Relying on algorithms for critical decisions, such as medical treatments or legal judgments, may erode individual independence. If a person cannot challenge or understand how a decision was made, they lose control over their own life choices.
Algorithmic accountability ensures a system of checks and balances to address these ethical concerns and hold parties responsible when moral standards are violated.
Potential Biases and Discrimination in Algorithms
Algorithms are only as good as the data on which they are trained. If the data reflects historical inequalities, the algorithm may reproduce and amplify these biases. This can result in unfair treatment of certain groups. Some common examples include:
- Racial Bias: Facial recognition technology has been shown to have higher error rates for people of color, leading to false identifications and potential wrongful arrests.
- Gender Bias: Recruitment algorithms may unintentionally favor male candidates over female candidates, especially in industries traditionally dominated by men.
- Socioeconomic Bias: Algorithms in loan approval processes may disadvantage individuals from lower socioeconomic backgrounds, even if they are otherwise qualified for a loan.
Ensuring accountability means regularly auditing algorithms for bias and taking corrective actions to prevent discriminatory outcomes.
Protecting Individual Rights and Ensuring Fairness
One of the core goals of algorithmic accountability is to protect the rights of individuals affected by algorithmic decisions. For example:
- Due Process: In legal contexts, individuals should be informed of and can challenge algorithmic decisions significantly when those decisions can negatively impact their lives (e.g., parole risk assessments or sentencing recommendations).
- Transparency: When algorithms are used in decision-making, individuals should understand the factors that influenced the outcome, allowing them to contest or appeal decisions.
- Fairness: Accountability mechanisms can help ensure that algorithms do not unfairly disadvantage certain groups, ensuring that all individuals are treated equitably.
Promoting fairness through accountability fosters trust in algorithmic systems and minimizes the risk of harm to individuals.
Promoting Trust in Automated Systems
Trust is essential for the successful adoption of any technology, especially when that technology makes decisions that directly affect people’s lives. People who feel they can trust algorithmic systems are more likely to accept and engage with them. Algorithmic accountability helps build trust by:
- Ensuring that decision-making processes are clear and understandable
- Providing avenues for redress if decisions are found to be unfair or harmful
- Demonstrating a commitment to ethical principles, such as non-discrimination and privacy protection
Without accountability, the public may view automated systems as “black boxes,” making it difficult for individuals to understand how decisions are made or to trust the outcomes.
Key Principles of Algorithmic Accountability
Transparency and Explainability in Algorithms
Transparency is one of the cornerstones of algorithmic accountability. It refers to the extent to which the workings of an algorithm can be understood by people who use or are affected by it. For algorithms to be transparent, they must be designed to allow both users and affected individuals to comprehend the decision-making process.
- Clear Decision Paths: Algorithms should be able to explain how they arrive at particular decisions, especially in high-stakes situations like legal judgments or healthcare diagnoses.
- Access to Information: Stakeholders, including consumers, regulators, and affected individuals, should be able to access relevant information about how algorithms function. This may include the data sources, the model used, and the factors influencing the decisions made.
Explainability complements transparency by ensuring that even non-experts can understand why an algorithm makes particular decisions. For example, if an algorithm is used in determining insurance premiums, explainability would ensure that individuals can understand how their premium was calculated and what factors contributed to it. Without transparency and explainability, the risk of unfairness and lack of trust increases.
Fairness and the Prevention of Bias
Ensuring fairness in algorithmic systems is vital to avoiding discriminatory outcomes. Fairness involves designing algorithms that treat all individuals and groups equitably, regardless of race, gender, socioeconomic status, or other protected characteristics.
- Bias Detection and Mitigation: Accountability requires regular audits to detect and address algorithm biases. For instance, if an algorithm is found to disproportionately disadvantage certain racial groups in loan approval processes, measures should be taken to adjust or retrain it.
- Bias Audits: Independent audits by third-party organizations can be valuable in assessing algorithmic fairness. These audits check whether the algorithm’s outputs are consistent with ethical standards, ensuring it does not inadvertently harm specific groups.
Algorithmic fairness extends beyond addressing explicit bias. It also involves minimizing implicit bias, which can be harder to detect but equally damaging. Fair algorithms ensure that all groups are treated equally, reducing the potential for systemic discrimination.
Responsiveness and Adaptability in Algorithms
Accountability also requires algorithms to be responsive and adaptable to new data and contexts. Algorithms should not be static once deployed; they must be regularly updated to reflect changing circumstances or new information.
- Continuous Monitoring: Algorithmic systems must be regularly monitored to ensure they remain accurate, unbiased, and aligned with their intended purposes. For instance, a predictive policing algorithm might need adjustments based on evolving crime data or shifts in public policy.
- Dynamic Feedback Loops: Systems should be designed to learn from their mistakes. If an algorithm produces an inaccurate or biased decision, the system should be capable of learning from that mistake and evolving. This dynamic adaptability ensures that algorithms remain relevant and responsible as circumstances change.
Without adaptability, algorithms may become outdated, ineffective, or potentially harmful over time. Regular updates, feedback loops, and continuous learning are essential for maintaining accountability.
Responsibility and Governance in Algorithmic Systems
Responsibility and governance are central to ensuring that algorithms are accountable for their actions. This involves clearly defined roles and processes for managing algorithms throughout their lifecycle, from development to deployment and beyond.
- Clear Ownership: Developers and organizations should clearly define who is responsible for the outcomes of algorithmic decisions. This includes establishing protocols for addressing any harm or errors caused by the algorithm.
- External Oversight: Independent bodies or regulatory agencies can provide oversight, ensuring that algorithms meet ethical standards and comply with relevant laws. External audits and evaluations offer an additional layer of accountability that prevents bias or neglect from going unnoticed.
Governance structures should include regular assessments, policy frameworks, and compliance checks to ensure algorithms are aligned with ethical, legal, and social standards. Clear accountability mechanisms allow the right parties to be held responsible when things go wrong, ensuring that the system works as intended and benefits society as a whole.
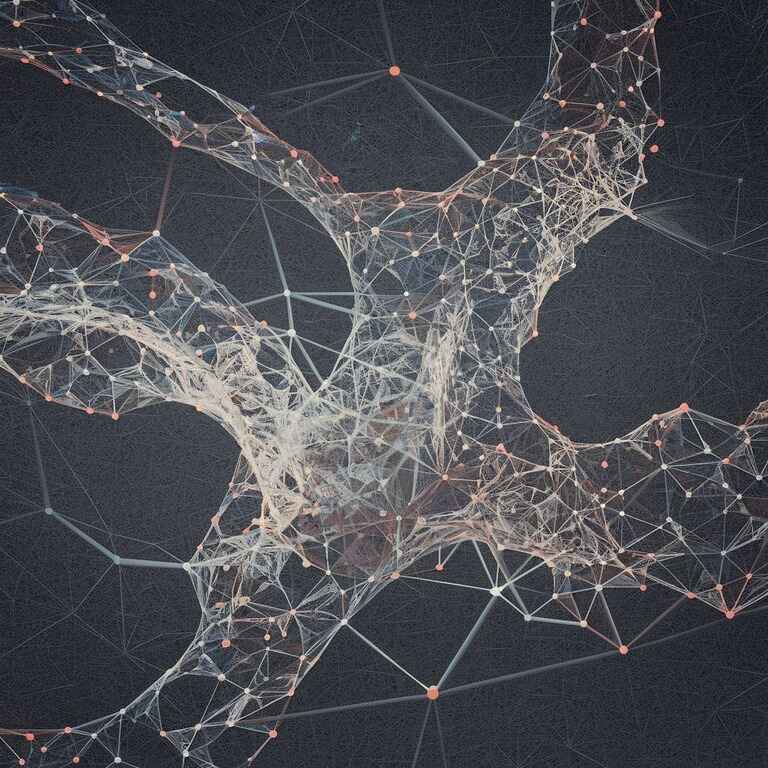
Challenges in Achieving Algorithmic Accountability
Complexity and Opacity in Advanced Algorithms
As algorithms become more sophisticated, they often grow more complex and less transparent. Many modern algorithms, particularly those based on machine learning and artificial intelligence, function as “black boxes” where it is difficult to trace how decisions are made. This complexity can make it challenging to determine how an algorithm processes data and arrives at its conclusions, hindering accountability efforts.
- Black Box Models: Machine learning models profound learning algorithms, can generate outcomes humans do not interpret easily. These models can learn patterns from data that are not immediately apparent, making it hard to explain their decision-making process.
- Lack of Traceability: When an algorithm fails or produces harmful outcomes, it can be difficult to trace the cause, especially if the model evolved based on changing data inputs. This opacity makes it harder to assign accountability to specific actions or entities.
Overcoming these challenges requires greater emphasis on designing algorithms that are effective and interpretable. Researchers and developers are working on creating more transparent AI systems, such as explainable AI (XAI), to address these issues.
Difficulty in Assessing and Addressing Biases
Even when an algorithm is transparent, identifying and addressing biases can be complex and ongoing. Biases may arise from various sources, including the data used to train the algorithm, the design of the algorithm itself, and the broader societal context in which the algorithm operates.
- Bias in Training Data: If the training data reflects historical biases or inequalities, the algorithm will likely perpetuate those biases. For example, a facial recognition system trained on predominantly white faces will perform poorly on people of color, leading to unfair outcomes.
- Hidden Biases: Algorithms may also exhibit biases that are not immediately visible or easy to quantify. These “hidden” biases may only emerge after the algorithm is deployed in the real world and subjected to diverse inputs and contexts.
Effectively addressing bias requires a multi-faceted approach, including data auditing, algorithm testing, and incorporating fairness principles into the algorithm’s design. Regular checks and iterative improvements are essential for mitigating bias over time.
The Challenge of Balancing Accountability with Innovation
One of the significant challenges in algorithmic accountability is balancing the need for accountability with the desire for innovation. Many new algorithms, particularly AI-driven, hold great promise in healthcare, transportation, and finance. However, these technologies often require much experimentation and flexibility to reach their full potential.
- Innovation Speed vs. Oversight: While algorithm developers are eager to push the boundaries of technology, regulatory bodies, and oversight mechanisms may slow down progress. Striking the right balance between ensuring accountability and fostering innovation is delicate.
- Regulation vs. Flexibility: Over-regulation can stifle creativity and delay the rollout of beneficial technologies. On the other hand, under-regulation can lead to unchecked risks, mainly when using algorithms in critical areas such as criminal justice or healthcare.
Finding a middle ground where accountability frameworks support innovation without stifling progress is essential for maintaining public trust and encouraging the responsible development of new technologies.
Legal and Regulatory Hurdles
The legal landscape surrounding algorithmic accountability is still evolving. While some countries and regions, such as the European Union, have introduced AI and data use regulations, many parts of the world lack comprehensive legal frameworks to govern algorithms. The challenges include:
- Global Inconsistencies: Different countries have different laws regarding data privacy, algorithmic transparency, and AI ethics. This creates uncertainty for international organizations, as they must navigate a patchwork of regulations.
- Regulatory Gaps: Many existing laws do not adequately address the challenges posed by modern algorithms, such as the risks of algorithmic bias or the accountability of automated decision-making systems. Updating legal frameworks to address these issues better is an ongoing challenge.
- Enforcement Issues: Even when regulations exist, enforcing them can be difficult, particularly in fast-moving industries where technological advancements outpace legal changes. Regulatory bodies must stay informed and adaptable to oversee algorithmic practices effectively.
To overcome these hurdles, policymakers must collaborate with technology experts, businesses, and civil society to create a robust legal framework that ensures accountability while fostering technological innovation.
Approaches to Enhancing Algorithmic Accountability
Establishing Ethical Guidelines and Standards
One effective way to enhance algorithmic accountability is to develop comprehensive ethical guidelines and industry standards. These guidelines can provide a roadmap for algorithm development and deployment, ensuring that they align with core ethical principles such as fairness, transparency, and privacy protection.
- Creating Industry Standards: Industry-wide standards can help set expectations for algorithmic accountability. These standards include guidelines for algorithm design, testing procedures, and transparency in decision-making. Companies can demonstrate their commitment to responsible AI use by adopting such standards.
- Ethical Review Boards: Some organizations have set up internal ethical review boards to assess the potential impact of algorithms before they are deployed. These boards evaluate whether the algorithm aligns with ethical guidelines and whether any unintended consequences could arise from its use.
Establishing and adhering to ethical guidelines helps ensure that algorithms are designed and used to benefit society while protecting individuals from harm.
Algorithm Auditing and Impact Assessments
Regular auditing and impact assessments are crucial tools in enhancing algorithmic accountability. These processes involve reviewing algorithms to evaluate their performance, fairness, and potential social impact before and after deployment.
- Algorithm Audits: Audits conducted by independent third-party organizations can assess whether algorithms function as intended and comply with ethical and legal standards. These audits may focus on bias, accuracy, and transparency.
- Impact Assessments: Before deploying an algorithm, businesses should conduct impact assessments to evaluate potential risks and harms. For example, in healthcare, an algorithm used for treatment recommendations might be assessed for its potential to harm vulnerable populations.
Algorithm auditing and impact assessments allow for early detection of issues and provide opportunities for corrective action, ensuring that algorithms remain accountable throughout their lifecycle.
Implementing Explainable AI (XAI) Technologies
As mentioned previously, one of the main challenges in algorithmic accountability is the opacity of many machine learning models. Explainable AI (XAI) technologies offer a solution by making algorithms more transparent and understandable.
- Improved Interpretability: XAI systems are designed to provide clear, human-readable explanations for their decisions, allowing users and affected individuals to understand the reasoning behind specific outcomes. For example, an AI system used to predict loan approvals might provide a breakdown of the factors influencing the decision, such as credit score or income.
- User-Friendly Explanations: The explanations provided by XAI models should be understandable to non-experts, allowing consumers and stakeholders to have greater confidence in the decision-making process and to challenge decisions when necessary.
By implementing XAI technologies, organizations can make their algorithms more accountable, ensuring users can trust the system’s decisions and feel empowered to question them.
Strengthening Legal and Regulatory Frameworks
Establishing robust legal and regulatory frameworks is essential to fostering algorithmic accountability. Effective regulations can set clear expectations for algorithmic behavior, enforce accountability, and provide mechanisms for redress when things go wrong.
- Data Protection and Privacy Laws: Regulations like the General Data Protection Regulation (GDPR) in the European Union have established important data privacy and transparency rules, offering valuable lessons for other regions looking to strengthen algorithmic accountability.
- Algorithmic Transparency Regulations: Some jurisdictions are beginning to introduce regulations specifically focused on algorithmic transparency. For example, laws requiring companies to disclose the use of automated decision-making systems in areas like hiring, credit scoring, or criminal justice can promote accountability.
- Liability and Redress Mechanisms: Liability must be defined in cases where algorithmic decisions result in harm or unfair outcomes. Clear redress mechanisms, including avenues for appeal and compensation, can help hold organizations accountable for their algorithms’ actions.
Stronger legal frameworks provide the necessary structure for holding developers and companies accountable for their algorithms, ensuring they align with ethical standards and protect individual rights.
Engaging Stakeholders and Promoting Public Awareness
Incorporating the voices of diverse stakeholders and raising public awareness are key steps in promoting algorithmic accountability. By involving a broad range of people in the conversation, developers can create more responsible algorithms that reflect society’s needs and values.
- Incorporating Public Feedback: Engaging the public in discussions about using algorithms in criminal justice, healthcare, and finance ensures that decisions are made with the broader community in mind. Feedback mechanisms such as public consultations or surveys can help identify potential concerns and improve algorithm design.
- Raising Awareness: Public awareness campaigns can educate consumers about their rights when interacting with algorithmic systems. When people understand how algorithms impact their lives, they are better equipped to demand transparency, fairness, and accountability from companies and governments.
By engaging stakeholders and fostering greater public awareness, organizations can create algorithms that are more accountable and more aligned with societal values.
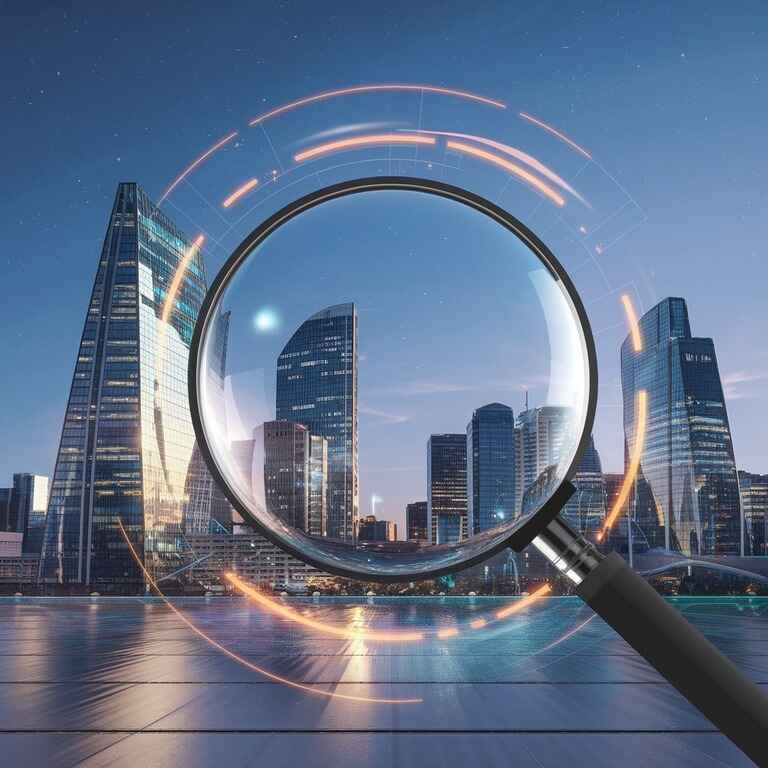
Future Directions in Algorithmic Accountability
Advancements in AI and Machine Learning for Accountability
As AI and machine learning technologies evolve, new tools and techniques will emerge to enhance algorithmic accountability. Future advancements in these fields hold the potential to address existing challenges and create more robust and transparent systems.
- AI-Driven Fairness Tools: New AI-driven tools are being developed to detect and mitigate bias in algorithms automatically. These tools can analyze large datasets to identify hidden biases and suggest adjustments to ensure fairer outcomes. For example, AI systems could monitor the decision-making processes in hiring algorithms to prevent discrimination based on gender or ethnicity.
- Enhanced Transparency through AI: As AI becomes more transparent, algorithms may become easier to interpret and understand. For instance, new AI models might integrate real-time monitoring features that allow users to see how specific data points influence algorithmic outcomes, making the system more interpretable and accountable.
These advancements in AI will help bridge the gap between complex machine learning models and the need for transparent, accountable decision-making, fostering greater trust in automated systems.
Global Collaboration on Algorithmic Accountability Standards
Given the tech industry’s global nature, establishing international standards for algorithmic accountability is necessary to ensure consistency and fairness across borders. Collaboration among countries, regulatory bodies, and technology firms can create shared guidelines and principles that promote ethical algorithm development.
- International Partnerships: Countries and organizations can form alliances to harmonize their approaches to algorithmic accountability. International collaboration on data privacy, transparency, and fairness can ensure that algorithms are held to the same ethical standards worldwide.
- Unified Regulatory Frameworks: The need for uniform regulatory frameworks for algorithmic accountability will grow as the global economy becomes increasingly interconnected. International regulators may develop standards that apply to global tech companies, ensuring they follow the same rules regardless of where they operate.
Global collaboration is essential for addressing the cross-border challenges posed by algorithms, such as data flows, privacy concerns, and the potential for algorithmic bias to spread across regions.
Emergence of Ethical AI Governance Models
The rise of AI and its pervasive influence across industries call for new governance models that prioritize ethical considerations alongside business objectives. Ethical AI governance involves integrating fairness, transparency, accountability, and privacy principles into every stage of the algorithm’s lifecycle.
- AI Ethics Committees: More companies are establishing AI ethics committees to oversee the development and deployment of algorithms. These committees can evaluate whether algorithms align with ethical principles and societal values, ensuring responsible decision-making.
- Decentralized Governance: In the future, we may see a shift toward decentralized AI governance, where multiple stakeholders, including developers, regulators, and affected individuals, share decision-making power. This could lead to more diverse perspectives in shaping algorithms’ design and implementation.
These governance models are critical to ensuring that AI is developed and deployed responsibly, focusing on mitigating risks and maximizing the benefits of these powerful technologies.
Increased Public and Consumer Advocacy for Accountability
As awareness of algorithmic accountability grows, we expect to see a stronger push from consumers and advocacy groups for greater transparency and fairness in algorithmic decision-making. Public pressure can drive companies and governments to prioritize accountability and develop more responsible algorithms.
- Consumer Rights Movements: As consumers become more aware of how algorithms impact their lives, there will be a greater demand for transparency and fairness. Advocacy groups will be key in pushing for stronger regulations and more responsible AI practices.
- Public Accountability Campaigns: Public campaigns highlighting unethical algorithmic practices, such as bias in hiring or criminal justice algorithms, can spur legislative action and influence corporate behavior. These campaigns can hold organizations accountable for using algorithms and demand better practices.
Increased public and consumer advocacy is likely to play a significant role in pushing for stronger algorithmic accountability, ensuring that the needs and rights of individuals are prioritized in the development of AI technologies.
Advancements in Algorithmic Regulation
The future of algorithmic accountability will also involve more sophisticated regulatory mechanisms that keep pace with technological advancements. As algorithms become more integrated into daily life, comprehensive regulation will be needed to ensure they are used responsibly.
- Adaptive Regulations: Regulators may develop more flexible, adaptive frameworks that can quickly respond to new developments in AI and machine learning. These regulations allow for continuous updates, ensuring algorithmic systems remain aligned with ethical standards as they evolve.
- Real-Time Monitoring and Auditing: Future regulatory models could involve real-time monitoring and auditing of algorithmic systems to ensure compliance with laws and ethical guidelines. Regulators could use AI-powered tools to track algorithmic behavior and detect potential violations, such as discrimination or unfair practices.
These advancements in regulatory mechanisms will play a crucial role in maintaining accountability and ensuring that algorithms continue to operate in ways that benefit society while minimizing harm.
Lorem ipsum dolor sit amet, consectetur adipiscing elit. Ut elit tellus, luctus nec ullamcorper mattis, pulvinar dapibus leo.
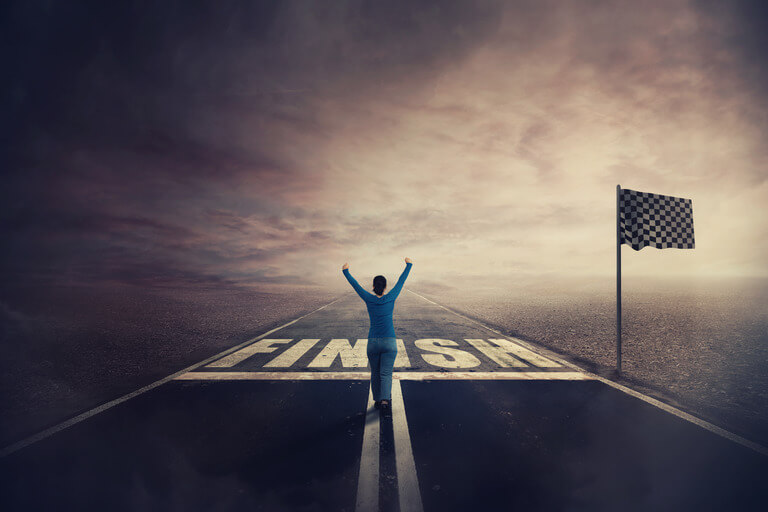
Breaking It All Down
Algorithmic accountability is a vital issue in today’s rapidly evolving technological landscape. As algorithms increasingly influence critical areas of life—from healthcare to criminal justice to employment—the need for transparent, fair, and responsible AI systems becomes more urgent. Addressing the challenges of complexity, bias, opacity, and regulation is essential for fostering public trust and ensuring that algorithms are used for the greater good.
While achieving full accountability presents numerous challenges, promising approaches and advancements hold the potential to make a significant impact. Ethical guidelines, algorithmic auditing, explainable AI technologies, and stronger legal frameworks are essential in ensuring algorithmic decision-making accountability. Furthermore, global collaboration and advancements in AI governance will help establish a shared standard for responsible algorithmic use.
As AI technology continues to develop, it is imperative that all stakeholders—governments, businesses, and the public—work together to create a future where algorithms are efficient, innovative but also accountable, and transparent. Through continued effort and collaboration, we can move toward a future where algorithms benefit society without compromising fairness, privacy, and ethical standards.
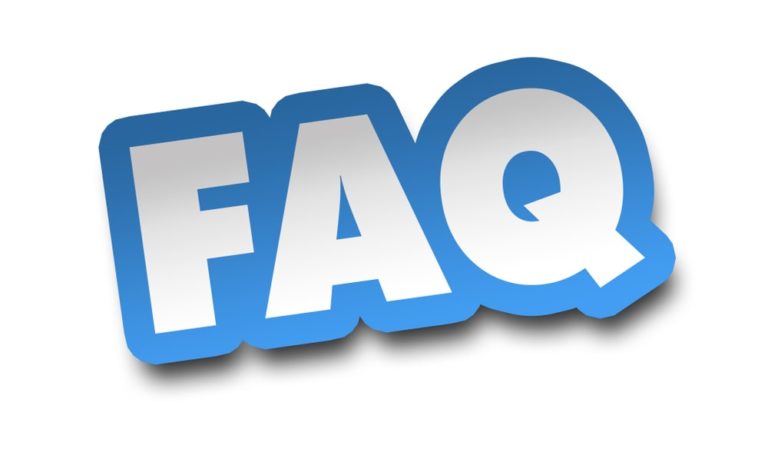
Frequently Asked Questions
What is algorithmic accountability?
Algorithmic accountability refers to the responsibility of developers, organizations, and governments to ensure that algorithms are designed, deployed, and used transparently, reasonably, and ethically. It involves being answerable for the outcomes generated by algorithms, including addressing issues like bias, transparency, and the potential for harm.
Why is algorithmic accountability necessary?
As algorithms influence more aspects of daily life, from hiring decisions to healthcare recommendations, ensuring these systems operate fairly, transparently, and without causing harm is critical. Accountability promotes trust in technology, helps prevent discrimination, and ensures that algorithms serve the public good.
What are the main challenges in achieving algorithmic accountability?
Some of the main challenges include:
- Complexity and opacity of advanced machine learning models.
- Bias in training data can lead to unfair outcomes.
- Lack of clear legal frameworks to regulate algorithmic systems.
The difficulty of balancing innovation with necessary oversight.
How can algorithmic accountability be improved?
Improvements can be made through:
- Establishing ethical guidelines and industry standards.
- Conducting algorithm audits and impact assessments to evaluate fairness and accuracy.
- Implementing explainable AI (XAI) to make algorithms more transparent.
- Strengthening legal frameworks to address algorithmic risks and ensure accountability.
Engaging stakeholders and raising public awareness of algorithmic impacts.
What is explainable AI (XAI)?
Explainable AI (XAI) refers to AI systems that provide human-readable explanations for their decisions and actions. This transparency allows users to understand how decisions are made, ensuring that algorithms are practical but also accountable and understandable.
How can bias in algorithms be addressed?
Bias in algorithms can be addressed by:
- Using diverse and representative training data to reduce biased outcomes.
- Conducting regular audits to identify and mitigate bias.
- Implementing algorithmic fairness techniques that focus on equitable outcomes for all users.
- Ensuring diverse perspectives are included in the design and evaluation of algorithms.
Are there any laws regulating algorithmic accountability?
Laws and regulations around algorithmic accountability are still evolving. The GDPR in the EU, for example, mandates transparency in automated decision-making and data privacy. Other regions, like the U.S., are working toward developing regulations around AI, bias, and fairness. However, comprehensive and global standards are still being developed.
Can public advocacy impact algorithmic accountability?
Public advocacy can drive change by raising awareness of unfair or discriminatory algorithmic practices. Advocacy groups and consumers pushing for greater transparency and fairness in AI can influence legislative action and encourage businesses to adopt more responsible algorithmic practices.
What role do businesses play in algorithmic accountability?
Businesses are responsible for ensuring that their algorithms are developed and used ethically. They can:
- Adhere to ethical guidelines and industry standards.
- Conduct regular algorithm audits and ensure transparency in decision-making.
- Use bias detection tools to ensure fairness in their algorithms.
Collaborate with regulatory bodies to follow legal and ethical standards.
How do global standards for algorithmic accountability work?
Global standards for algorithmic accountability involve international collaboration to establish common principles and guidelines that tech companies must follow, regardless of the country in which they operate. These standards aim to ensure that algorithms are used ethically, protect privacy, and avoid discrimination while allowing for innovation.
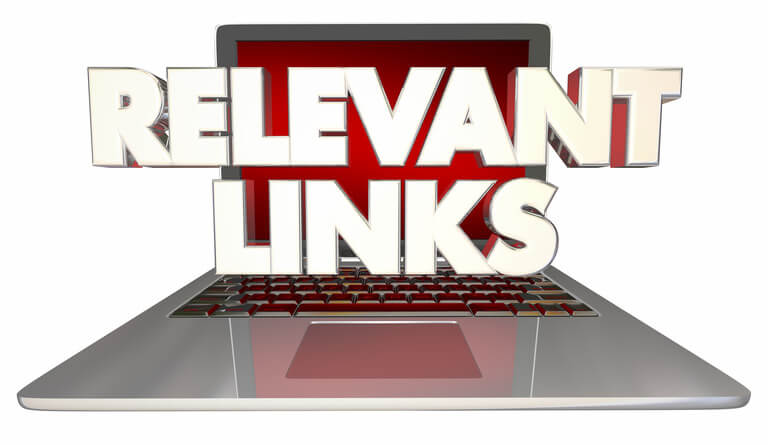
- Criminal Defense Attorneys: Learn about criminal defense services offered by our experienced attorneys in Las Vegas.
- Las Vegas DUI Lawyer: Get experienced legal representation if you’re facing DUI charges in Las Vegas.
- Domestic Violence Lawyer Las Vegas: Find out how our attorneys can help with domestic violence cases in Las Vegas.
- Drug Possession Lawyer: Our attorneys specialize in defending clients facing drug possession charges.
- Sex Crimes Attorney: We offer strong defense strategies for individuals accused of sex crimes in Las Vegas.
- CPS Defense Attorney: Defense for those facing child abuse or neglect allegations in Nevada.
- Misdemeanor Lawyer: Our attorneys provide adequate defense for misdemeanor charges in Las Vegas.
- Las Vegas Warrant Defense Attorney: Legal help for individuals facing arrest warrants in Las Vegas.
- Las Vegas Probation Violation Attorney: Learn how we can assist with probation violations in Las Vegas.
- Theft Crime Defense Lawyer: Get legal representation for theft-related charges in Las Vegas.
- Kidnapping Lawyers: Experienced lawyers defending individuals accused of kidnapping.
- Juvenile Defense Lawyers: Specialized legal defense for juveniles facing criminal charges.
- Firearms Lawyer Las Vegas: Legal representation for those charged with firearm offenses in Las Vegas.
These resources provide detailed information and guidance for anyone facing criminal charges in Nevada.
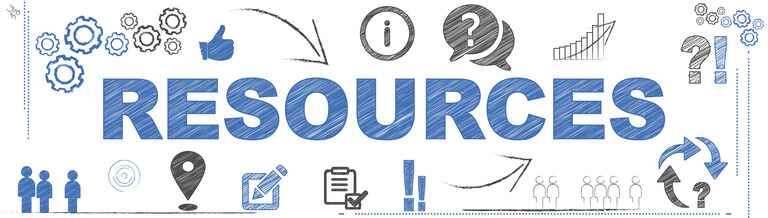
Offsite Resources for You
- AI Now Institute
- A research institute focused on studying the social implications of AI, emphasizing the importance of accountability and fairness in algorithmic decision-making.
- Algorithmic Justice League
- A nonprofit organization advocating for ethical AI development, working to eliminate bias and promote accountability within algorithmic systems.
- Partnership on AI
- An organization committed to advancing AI technologies in a way that ensures their ethical use, including a focus on accountability in AI systems across industries.
- Montreal AI Ethics Institute
- A leading think tank dedicated to addressing the ethical, social, and political issues associated with AI and machine learning, including algorithmic accountability.
- Fairness, Accountability, and Transparency (FAT*)
- A conference and community dedicated to advancing research and dialogue on fairness, accountability, and transparency in AI and machine learning systems.
- Data & Society
- A research organization studying the impact of data-centric technologies on society, including the ethical and accountability issues surrounding algorithmic decision-making.
- These organizations and resources provide extensive information and research on algorithmic accountability, offering a broader context for understanding its significance and the ongoing work in the field.
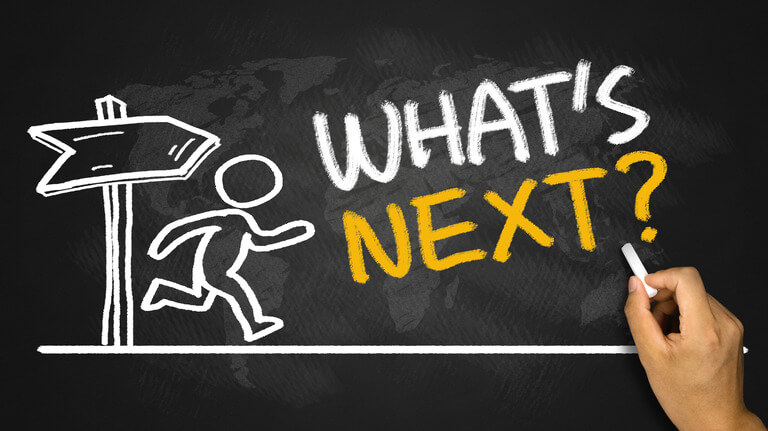
A Special Message from Our Lead Attorney, Molly Rosenblum Allen, Esq
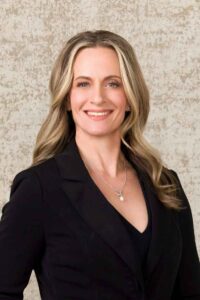
Thank you for taking the time to explore these resources. I hope they helped you better understand algorithmic accountability and its impact. If you’re facing a situation that requires legal assistance, my team and I are here to help. Feel free to call us at (702) 433-2889 to get the ball rolling on your case. We’re ready to assist you in navigating your legal needs.
Best regards,
Molly Rosenblum Allen, Esq.
The Rosenblum Allen Law Firm